Py: K-means clustering of COVID dataset#
This notebook was originally created by Amanda Aitken for the Data Analytics Applications subject, as Exercise 6.4 - K-means clustering of COVID dataset, in the DAA M06 Unsupervised learning module.
Data Analytics Applications is a Fellowship Applications (Module 3) subject with the Actuaries Institute that aims to teach students how to apply a range of data analytics skills, such as neural networks, natural language processing, unsupervised learning and optimisation techniques, together with their professional judgement, to solve a variety of complex and challenging business problems. The business problems used as examples in this subject are drawn from a wide range of industries.
Find out more about the course here.
Purpose#
The following code performs K-means clustering on COVID data. Once you have read through the code, run it and inspected the output, you should try using different values of K and observe the differences in the clustering outcomes.
References#
The dataset that is used in this exercise was sourced from Our World in Data at https://ourworldindata.org/covid-cases.
This dataset was downloaded from the above link on 31 March 2021. It contains country-by-country data on confirmed coronavirus disease (COVID-19) cases and at the time of writing is updated on a daily basis.
The data contains COVID-19 and population related features for over 100 countries. These features include:
total cases per million people;
total new cases per million people;
total deaths per million people;
new deaths per million people;
reproduction rate of the disease;
positive testing rate;
total tests per thousand people;
icu patients per million people; and
hospital patients per million people.
Packages#
This section installs packages that will be required for this exercise/case study.
import pandas as pd # Used for data management.
import matplotlib.pyplot as plt
%matplotlib inline
# The following scikit-learn libraries will be used
# to standardise the features and run K-means clustering.
from sklearn.pipeline import make_pipeline
from sklearn.preprocessing import StandardScaler
from sklearn.cluster import KMeans
Data#
This section:
imports the data that will be used in the modelling;
explores the data; and
prepares the data for modelling.
Import data#
# Note that the following code could be used to read the most
# recent data in directly from the Our World in Data website:
# covid = pd.read_csv('https://covid.ourworldindata.org/data/owid-covid-data.csv')
# However, we will use a snapshot so that the notebook keeps working even if the dataset format changes.
# Create a dataset called 'covid'.
covid = pd.read_csv('https://actuariesinstitute.github.io/cookbook/_static/daa_datasets/DAA_M06_COVID_data.csv.zip', header = 0)
Prepare data#
# Restrict the data to only look at one point in time (31-Dec-2020)
covid2 = covid[covid['date']=='2020-12-31']
# This analysis will use nine features in the clustering.
# The column 'location' is also retained to give us the country names.
# Countries that have missing values at the extract date are dropped from
# the data table using the .dropna() method.
covid3 = covid2[['location','total_cases_per_million','new_cases_per_million',
'total_deaths_per_million','new_deaths_per_million',
'reproduction_rate','positive_rate','total_tests_per_thousand',
'icu_patients_per_million','hosp_patients_per_million']].dropna()
covid_data = covid3.drop(columns='location')
print(covid_data.info())
countries = covid3['location'].tolist()
print(countries)
<class 'pandas.core.frame.DataFrame'>
Int64Index: 17 entries, 4823 to 74527
Data columns (total 9 columns):
# Column Non-Null Count Dtype
--- ------ -------------- -----
0 total_cases_per_million 17 non-null float64
1 new_cases_per_million 17 non-null float64
2 total_deaths_per_million 17 non-null float64
3 new_deaths_per_million 17 non-null float64
4 reproduction_rate 17 non-null float64
5 positive_rate 17 non-null float64
6 total_tests_per_thousand 17 non-null float64
7 icu_patients_per_million 17 non-null float64
8 hosp_patients_per_million 17 non-null float64
dtypes: float64(9)
memory usage: 1.3 KB
None
['Austria', 'Belgium', 'Bulgaria', 'Canada', 'Cyprus', 'Denmark', 'Estonia', 'Finland', 'Ireland', 'Israel', 'Italy', 'Luxembourg', 'Portugal', 'Slovenia', 'Spain', 'United Kingdom', 'United States']
Modelling#
Fit model#
This section performs K-means clustering.
# Perform K-means clustering on the COVID data.
# Create a scaler so that the features in the dataset can be
# scaled to have a mean of 0 and a standard deviation of 1.
scaler = StandardScaler()
# Create a KMeans model with k clusters.
# You can experiment with different values of k here.
k = 3
kmeans = KMeans(n_clusters=k)
# Create a pipeline to link together the scaler and kmeans instance.
pipeline = make_pipeline(scaler,kmeans)
# Build a K-means clustering model by fitting the pipeline to the COVID dataset.
pipeline.fit(covid_data)
# Predict the cluster labels for the COVID dataset.
labels = pipeline.predict(covid_data)
# Create a DataFrame, df, aligning labels and countries.
df = pd.DataFrame({'labels': labels, 'countries': countries})
# Display df sorted by cluster label.
print(df.sort_values(by='labels'))
labels countries
11 0 Luxembourg
0 1 Austria
14 1 Spain
13 1 Slovenia
12 1 Portugal
10 1 Italy
15 1 United Kingdom
16 1 United States
2 1 Bulgaria
1 1 Belgium
6 2 Estonia
9 2 Israel
5 2 Denmark
4 2 Cyprus
3 2 Canada
7 2 Finland
8 2 Ireland
Plot elbow curve#
One method of selecting an appropriate value for K is to plot a graph of the within-cluster sum of squares, ๐๐ถ๐๐ or inertia, for different values of K.
Elbow curves are described in Module 6.
# Calculate the WCSS or inertia for different values of K.
WCSS = []
K = range(1,10)
for k in K:
kmeans2 = KMeans(n_clusters=k)
pipeline2 = make_pipeline(scaler,kmeans2)
pipeline2.fit(covid_data)
WCSS.append(kmeans2.inertia_)
# Plot the elbow curve. ('bx-')
plt.figure(figsize=(16,8))
plt.plot(K, WCSS,color='dodgerblue')
plt.xlabel('k')
plt.ylabel('WCSS')
plt.title('Elbow curve for the COVID data')
plt.show()
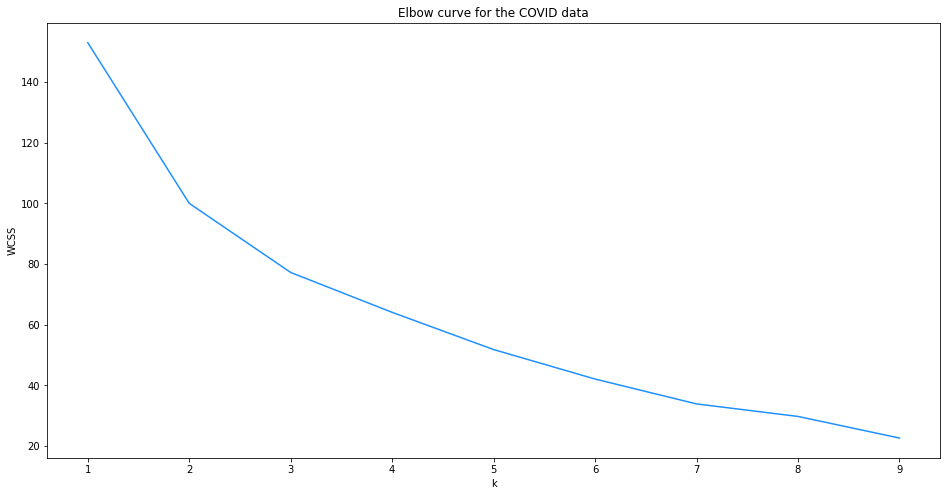
The elbow curve suggests that a selection of K = 2 might be appropriate for this data as there is a kink in the curve at this point. However, you could also argue that a selection of K = 4 might be more appropriate because it results in a lower within cluster sum of squares (WCSS) and there is also a slight kink in the plot at this point.