Py: Socio-Economic Index Construction#
This notebook was originally created by Andres Villegas Ramirez for the Data Analytics Applications subject, as Case study 1 - The Socio-Economic Indexes for Areas in the DAA M06 Unsupervised learning module.
Data Analytics Applications is a Fellowship Applications (Module 3) subject with the Actuaries Institute that aims to teach students how to apply a range of data analytics skills, such as neural networks, natural language processing, unsupervised learning and optimisation techniques, together with their professional judgement, to solve a variety of complex and challenging business problems. The business problems used as examples in this subject are drawn from a wide range of industries.
Find out more about the course here.
Purpose:#
This case study demonstrates the use of principal component analysis (PCA) in the construction of an index to rank the socio-economic status of different geographical areas within a country (in this instance, Australia). Such index can be useful to identify areas that require funding or services, or as input for research into the relationship between socio-economic conditions and other outcomes in different areas.
In Australia, the Australian Bureau of Statistics (ABS) produces four of such indexes, referred to as the Socio-Economic Indexes for Areas (SEIFA). SEIFA ranks areas in Australia based on their relative socio-economic advantage and disadvantage.
This notebook replicates the methodology adopted by the ABS in constructing one of these indexes - Index of Economic Resources, or IER by Statistical Area 1 (SA1).
This Jupyter notebook is organised into the following sections:#
Section 1: Load required packages
Section 2: Define a function for plotting
Section 3: Import and transform data
Section 4: Apply Principal Component Analysis and calibrate SEIFA score
Section 5: Check against ABS publication
1. Load Packages#
# Import required Python packages
#Dataframe operations
import pandas as pd
#PCA
from sklearn.decomposition import PCA
from sklearn.preprocessing import StandardScaler
#Plotting
import matplotlib.pyplot as plt
2. Define Functions#
# Define a function to obtain the explained variances from the PCA features.
## Good practice to define functions upfront.
def plot_explained_variances(pca):
exp_var_pca = pca.explained_variance_ratio_
plt.bar(range(1, len(exp_var_pca) + 1), exp_var_pca, alpha = 0.5, align = 'center',
label='Individual explained variance',color='dodgerblue')
plt.ylabel('Explained variance ratio')
plt.xlabel('Principal component')
plt.legend(loc='best')
plt.tight_layout()
plt.show()
3. Import and Transform Data#
# Import data
## File 1: Standardised socio-economic variables published by the ABS (i.e. input to the PCA model)
## File 2: IER scores by Statistical Area 1 published by the ABS
infolder = 'https://actuariesinstitute.github.io/cookbook/_static/daa_datasets/'
file1 = 'standardised_variables_seifa_2021_cut.csv'
file2 = 'IER_2021.csv'
data1 = pd.read_csv(infolder + file1)
data2 = pd.read_csv(infolder + file2)
# Inspect data
## These are numerical features (e.g. % corresponding to a variable, see dictionary below) which are standardised
data1.head()
SA1_2021 | INC_LOW | INC_HIGH | ATSCHOOL | ATUNI | CERTIFICATE | DEGREE | DIPLOMA | NOYR12ORHIGHER | NOEDU | ... | HIGHCAR | NOCAR | DISABILITYU70 | ENGLISHPOOR | ONEPARENT | SEP_DIVORCED | UNINCORP | CHILDJOBLESS | MORTGAGEOVER30 | RENTOVER30 | |
---|---|---|---|---|---|---|---|---|---|---|---|---|---|---|---|---|---|---|---|---|---|
0 | 10102100701 | 0.031160 | -0.451226 | -0.307998 | -0.611843 | 2.110423 | -0.792820 | 1.399224 | 0.039552 | -0.494326 | ... | 1.124942 | -0.361674 | 1.160679 | -0.628432 | -0.087881 | -0.046096 | 0.533110 | -0.797863 | 0.427678 | -1.208146 |
1 | 10102100702 | -0.745478 | 0.235597 | 0.309750 | -0.718416 | 0.795239 | -0.138695 | 1.122862 | -0.328068 | -0.494326 | ... | 2.165534 | -0.737816 | -1.371078 | -0.628432 | -1.513208 | -0.216841 | 1.846095 | -0.797863 | 0.322564 | -1.208146 |
2 | 10102100703 | 0.207462 | -0.333816 | 0.648522 | -0.373289 | 0.143017 | 0.089753 | -0.024178 | -0.148292 | -0.494326 | ... | -0.474119 | -0.425240 | -1.064640 | -0.628432 | 0.078716 | 0.963607 | 0.486319 | -0.335393 | -0.486090 | -0.029516 |
3 | 10102100704 | 0.383411 | -0.417133 | -1.082034 | -0.887458 | -0.308372 | 0.417513 | -0.160872 | 0.083635 | 0.108973 | ... | -0.407742 | -0.528653 | 0.038035 | -0.628432 | 0.949299 | 1.482164 | 0.808398 | 0.554274 | -0.156349 | -0.423796 |
4 | 10102100705 | 0.334499 | 0.256282 | -1.018268 | -0.396676 | 0.235498 | -0.072269 | -0.227208 | 0.356362 | -0.494326 | ... | -0.135464 | -0.477578 | 0.056221 | -0.628432 | -1.048310 | 1.253986 | 0.795448 | -0.116016 | -0.297469 | -0.292223 |
5 rows × 45 columns
Variable dictionary#
Variable name |
Variable description |
---|---|
INC_LOW |
Per cent of people living in households with stated annual household equivalised income between \(1 and \)25,999 (approx. 1st and 2nd deciles) |
LOWRENT |
Per cent of occupied private dwellings paying rent less than \(250 per week (excluding \)0 per week) |
NOCAR |
Per cent of occupied private dwellings with no cars |
LONE |
Per cent of occupied private dwellings who are lone person occupied private dwellings |
ONEPARENT |
Per cent of one parent families with dependent offspring only |
OVERCROWD |
Per cent of occupied private dwellings requiring one or more extra bedrooms (based on Canadian National Occupancy Standard) |
UNEMPLOYED_IER |
Per cent of people aged 15 years and over who are unemployed |
GROUP |
Per cent of occupied private dwellings who are group occupied private dwellings |
OWNING |
Per cent of occupied private dwellings owning dwelling without a mortgage |
UNINCORP |
Per cent of dwellings with at least one person who is an owner of an unincorporated enterprise |
INC_HIGH |
Per cent of people with stated annual household equivalised income greater than $91,000 |
HIGHMORTGAGE |
Per cent of occupied private dwellings paying mortgage greater than $2,800 per month |
MORTGAGE |
Per cent of occupied private dwellings owning dwelling (with a mortgage) |
HIGHBED |
Per cent of occupied private dwellings with four or more bedrooms |
# Inspect data
## IER scored published based on 2021 Census data collection
data2.head()
SA1_2021 | IER_2021 | |
---|---|---|
0 | 10102100701 | 1023.037282 |
1 | 10102100702 | 1088.576036 |
2 | 10102100703 | 986.1022032 |
3 | 10102100704 | 965.4964701 |
4 | 10102100705 | 1013.432808 |
# Select variables ABS uses to calibrate the IER (per their methodology)
variable_IER = [
'SA1_2021',
'INC_LOW',
'LOWRENT',
'NOCAR',
'LONE',
'ONEPARENT',
'OVERCROWD',
'UNEMPLOYED_IER',
'GROUP',
'OWNING',
'UNINCORP',
'INC_HIGH',
'HIGHMORTGAGE',
'MORTGAGE',
'HIGHBED'
]
data1_IER = data1[variable_IER]
# Inspect data type for these variables
data1_IER.info()
<class 'pandas.core.frame.DataFrame'>
RangeIndex: 59430 entries, 0 to 59429
Data columns (total 15 columns):
# Column Non-Null Count Dtype
--- ------ -------------- -----
0 SA1_2021 59430 non-null int64
1 INC_LOW 59427 non-null float64
2 LOWRENT 59400 non-null float64
3 NOCAR 59422 non-null float64
4 LONE 59430 non-null float64
5 ONEPARENT 59319 non-null float64
6 OVERCROWD 59417 non-null float64
7 UNEMPLOYED_IER 59429 non-null float64
8 GROUP 59430 non-null float64
9 OWNING 59423 non-null float64
10 UNINCORP 59430 non-null float64
11 INC_HIGH 59427 non-null float64
12 HIGHMORTGAGE 59400 non-null float64
13 MORTGAGE 59423 non-null float64
14 HIGHBED 59422 non-null float64
dtypes: float64(14), int64(1)
memory usage: 6.8 MB
# Remove rows with missing values
print(data1_IER.isna().sum())
data1_IER_dropna = data1_IER.dropna()
SA1_2021 0
INC_LOW 3
LOWRENT 30
NOCAR 8
LONE 0
ONEPARENT 111
OVERCROWD 13
UNEMPLOYED_IER 1
GROUP 0
OWNING 7
UNINCORP 0
INC_HIGH 3
HIGHMORTGAGE 30
MORTGAGE 7
HIGHBED 8
dtype: int64
# Standardise data for PCA
## Select data from column 2 onwards (inclusive). First column is SA1 ID.
pca_data = data1_IER_dropna.iloc[:, 1:]
## Standardise these columns to mean 0 and variance 1
sc = StandardScaler()
sc.fit(pca_data)
pca_data = sc.transform(pca_data)
## Inspect outputs, each row represents a SA1
print(data1_IER_dropna[50:55])
print(pca_data[50:55])
SA1_2021 INC_LOW LOWRENT NOCAR LONE ONEPARENT OVERCROWD \
50 10102100921 -0.694978 -0.149470 0.026395 2.161618 0.016894 0.614584
51 10102100923 -0.399570 -0.230663 -0.392438 0.346039 0.479932 -0.327006
53 10102100925 -1.184584 -0.369694 -0.584525 1.995606 1.010923 0.163947
54 10102100926 -0.755434 0.636737 0.194704 1.770099 -0.418760 0.828480
55 10102100927 -0.534872 -0.113768 -0.443336 -0.248692 -0.929502 0.042116
UNEMPLOYED_IER GROUP OWNING UNINCORP INC_HIGH HIGHMORTGAGE \
50 -0.768433 -0.298797 -1.379936 -1.409706 0.565708 -0.711816
51 -0.502225 -0.435782 -0.068099 -1.078581 0.417556 -0.309550
53 0.393123 0.492720 -1.552475 -0.869567 0.401089 -0.914661
54 0.562322 1.018832 -1.283807 -0.853606 -0.501616 -0.914661
55 -0.591322 0.001803 -0.652794 -0.622710 0.248079 0.145029
MORTGAGE HIGHBED
50 -0.075949 -1.407022
51 0.780225 0.198026
53 -0.382142 -1.473097
54 -1.468772 -1.443632
55 0.547895 0.465557
[[-6.97384349e-01 -1.49102654e-01 2.73842498e-02 2.18456511e+00
1.68271418e-02 6.21443888e-01 -7.82538918e-01 -3.01193486e-01
-1.38687638e+00 -1.42934823e+00 5.65712045e-01 -7.14208804e-01
-8.01255787e-02 -1.41014150e+00]
[-4.00578528e-01 -2.30600290e-01 -3.93807726e-01 3.52373086e-01
4.80225191e-01 -3.29406608e-01 -5.11392783e-01 -4.39108873e-01
-7.01834243e-02 -1.09373813e+00 4.17385805e-01 -3.11130864e-01
7.79986710e-01 1.96337876e-01]
[-1.18930828e+00 -3.70152778e-01 -5.86976088e-01 2.01703428e+00
1.01162817e+00 1.66375413e-01 4.00564091e-01 4.95697733e-01
-1.56005434e+00 -8.81893199e-01 4.00899556e-01 -9.17462999e-01
-3.87727179e-01 -1.47627516e+00]
[-7.58127412e-01 6.40055426e-01 1.96640397e-01 1.78946385e+00
-4.19164718e-01 8.37443911e-01 5.72902051e-01 1.02538267e+00
-1.29039226e+00 -8.65715951e-01 -5.02866086e-01 -9.17462999e-01
-1.47935650e+00 -1.44678433e+00]
[-5.36520854e-01 -1.13266264e-01 -4.44992083e-01 -2.47800227e-01
-9.30303140e-01 4.33461879e-02 -6.02142493e-01 1.44766450e-03
-6.57042865e-01 -6.31693191e-01 2.47710087e-01 1.44364950e-01
5.46588117e-01 4.64107182e-01]]
4. Modelling#
This section performs the PCA to recreate the ABS’s IER Index.
# Initiate the PCA.
pca = PCA()
# Fit the PCA model to determine the transformed features.
## It took 2 lines to fit the PCA model.
pca.fit_transform(pca_data)
# Plot variance explained by principal components
plot_explained_variances(pca)
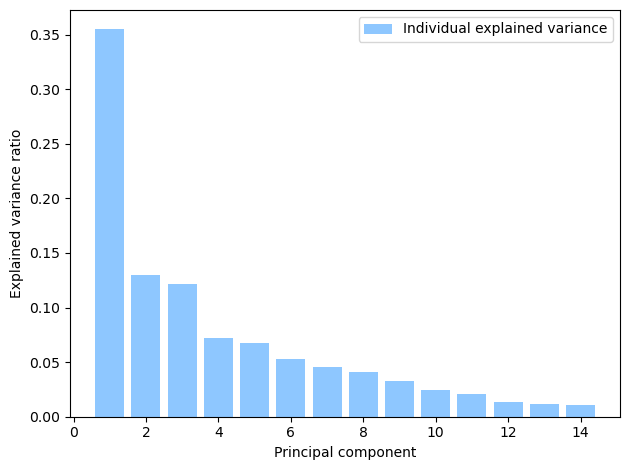
Principal component 1 (PC1) explains over 30% of the variance in the data. PC1 is used as the basis of calculating the IER.
pca_data[0]
array([ 3.21902216e-02, -1.95351071e-01, -3.62869762e-01, 3.59364682e-01,
-8.80285299e-02, 1.99924572e-01, -6.52078395e-01, -9.12914962e-01,
1.26794033e+00, 5.39782081e-01, -4.52416487e-01, -3.17209382e-01,
2.45495526e-01, 4.39423025e-04])
# Reverse sign (per ABS methodology)
pca_data_transformed = -1.0*pca.fit_transform(pca_data)
# Extract first principal component in a dataframe
pca1 = pd.DataFrame(pca_data_transformed[:, 0], columns = ['IER_2021'])
# Attach SA1 ID to
IER_S1 = pd.concat([data1_IER_dropna['SA1_2021'].reset_index(drop = True), pca1]
, axis=1)
print(IER_S1.head(1))
SA1_2021 IER_2021
0 10102100701 0.517295
# Calculation from first principal
## Sign implies how each variable contributes to a score
## Reverse sign to make it more comprehensive
component_df = pd.DataFrame({
'Variable': data1_IER.columns[1:],
'PC1 Loading': -1.0 * pca.components_[0],
'First SA1': pca_data[0]
})
print(component_df)
raw_score_first_SA1 = (component_df['PC1 Loading'] * component_df['First SA1']).sum()
print('\n')
print('\n')
print(raw_score_first_SA1)
Variable PC1 Loading First SA1
0 INC_LOW -0.327920 0.032190
1 LOWRENT -0.320014 -0.195351
2 NOCAR -0.316516 -0.362870
3 LONE -0.307799 0.359365
4 ONEPARENT -0.243785 -0.088029
5 OVERCROWD -0.231555 0.199925
6 UNEMPLOYED_IER -0.217694 -0.652078
7 GROUP -0.175738 -0.912915
8 OWNING 0.152087 1.267940
9 UNINCORP 0.211586 0.539782
10 INC_HIGH 0.233597 -0.452416
11 HIGHMORTGAGE 0.285636 -0.317209
12 MORTGAGE 0.295868 0.245496
13 HIGHBED 0.334995 0.000439
0.5172947202278048
A recap of the dataframe operations for transforming raw data to principal components#
Dataframe name |
Operations |
---|---|
data1 |
Raw data we’ll be using to replicate the SEIFA IER score, numercial features (%) |
data1_IER |
Select features for determining IER from data1 |
data1_IER_dropna |
Remove missing values in data1_IER |
pca_data |
Standardise features in data1_IER_dropna to mean 0 and s.d. 1, used to perform PCA |
pca |
Output of PCA, Principal Components |
pca_data_transformed |
Reverse sign in pca |
pca1 |
Extract first principal component from pca_data_transformed |
IER_S1 |
Attach SA1 identifier to pca1, compared to dataframe ABS_IER_S1 which is the published data |
# Standardise calibrated IER scores to mean of 1,000 and standard deviation of 100 (per ABS methodology)
IER_S1['IER_recreated'] = (IER_S1['IER_2021']/IER_S1['IER_2021'].std())*100+1000
print(IER_S1.tail())
SA1_2021 IER_2021 IER_recreated
59298 90104100401 0.022187 1000.995399
59299 90104100402 -0.055935 997.490510
59300 90104100403 -0.785740 964.748521
59301 90104100404 -1.702813 923.604956
59302 90104100407 -0.116936 994.753789
5. Reconcile with ABS publication#
# Extract first two columns in the desired data type
ABS_IER_S1 = data2.iloc[:, [0, 1]]
ABS_IER_S1['IER_2021'] = pd.to_numeric(ABS_IER_S1['IER_2021'],
errors='coerce')
ABS_IER_S1['SA1_2021'] = pd.to_numeric(ABS_IER_S1['SA1_2021'],
errors='coerce', downcast = 'integer')
print(ABS_IER_S1.info())
print('\n')
print(ABS_IER_S1.head())
print('\n')
print(ABS_IER_S1.isna().sum())
<class 'pandas.core.frame.DataFrame'>
RangeIndex: 59421 entries, 0 to 59420
Data columns (total 2 columns):
# Column Non-Null Count Dtype
--- ------ -------------- -----
0 SA1_2021 59421 non-null int64
1 IER_2021 59303 non-null float64
dtypes: float64(1), int64(1)
memory usage: 928.6 KB
None
SA1_2021 IER_2021
0 10102100701 1023.037282
1 10102100702 1088.576036
2 10102100703 986.102203
3 10102100704 965.496470
4 10102100705 1013.432808
SA1_2021 0
IER_2021 118
dtype: int64
## Remove rows with missing value
print(ABS_IER_S1.isna().sum())
ABS_IER_S1_dropna = ABS_IER_S1.dropna()
print(len(data1_IER_dropna))
print(len(ABS_IER_S1_dropna))
SA1_2021 0
IER_2021 118
dtype: int64
59303
59303
# Merge the recreated with the published data for reconciliation 1 - histograms
join = pd.merge(ABS_IER_S1_dropna, data1_IER_dropna, how = 'left', on = 'SA1_2021')
# Plot histogram of calibrated IER scores
IER_S1.hist(column='IER_recreated', bins=100, color='dodgerblue')
plt.title('Distribution of recreated IER scores')
plt.xlabel('IER score')
plt.ylabel('Frequency')
plt.show()
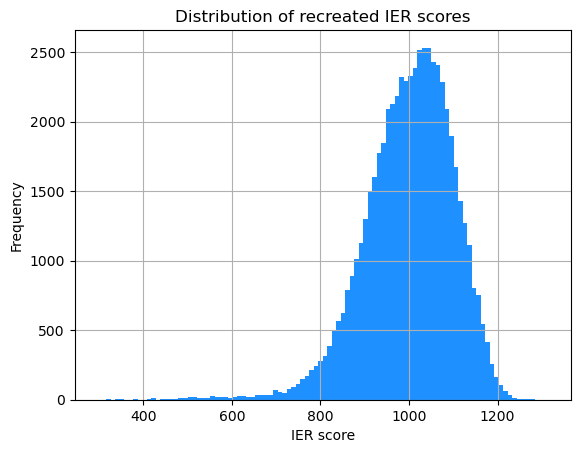
# Plot histogram of publisehd IER scores
ABS_IER_S1_dropna.hist(column='IER_2021', bins=100, color='dodgerblue')
plt.title('Distribution of ABS IER scores')
plt.xlabel('IER score')
plt.ylabel('Frequency')
plt.show()
ABS_IER_S1_dropna.to_csv('check_ABS_IER_S1_dropna.csv')
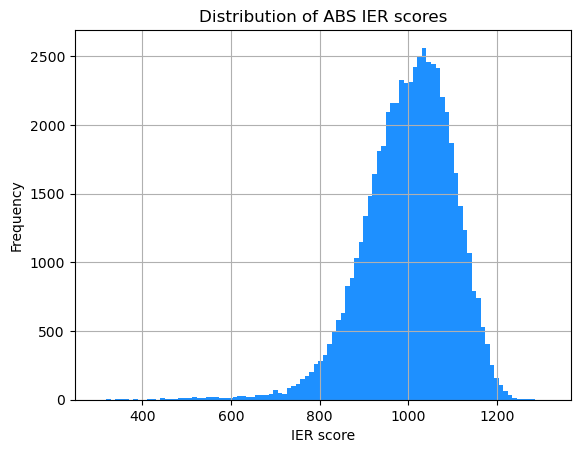
The two histograms are very similar in shape!
# Extract columns we need
IER_S1_reduce = IER_S1[['SA1_2021', 'IER_recreated']]
# Merge the recreated with the published data for reconciliation 2 - scatter plot
IER_join = pd.merge(ABS_IER_S1_dropna, IER_S1_reduce, how = 'left', on = 'SA1_2021')
print(IER_join.tail())
SA1_2021 IER_2021 IER_recreated
59298 90104100401 1000.369129 1000.995399
59299 90104100402 996.979765 997.490510
59300 90104100403 964.139169 964.748521
59301 90104100404 923.050144 923.604956
59302 90104100407 994.377312 994.753789
# Plot calibrated score vs. published score
plt.scatter('IER_recreated', 'IER_2021', data = IER_join, color='dodgerblue')
plt.title('Comparison of recreated and ABS IER scores')
plt.xlabel('Recreated IER score')
plt.ylabel('ABS IER score')
plt.show()
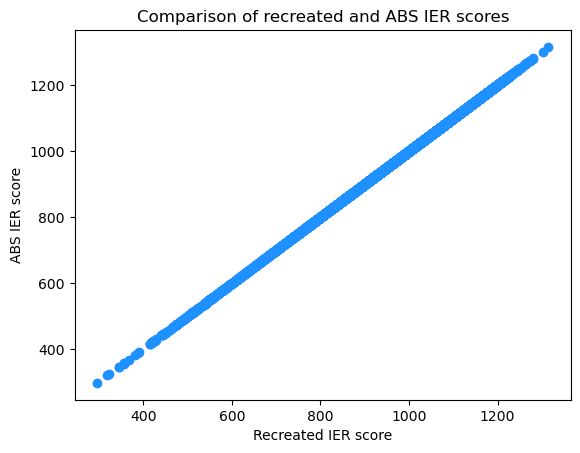
The above scatter plot shows that the two sets of indexes are very closely aligned.